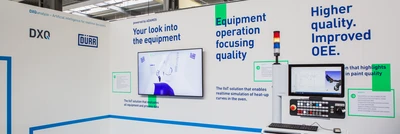
Artifical intelligence for realtime decisions
Oct 21, 2019
What DXQanalyze stands for
DXQanalyze is one of the four product families of our Digital Factory. The family includes the analysis software DXQequipment.analytics, which records all data during the painting process as well as during the entire car body drying process. As a further member of DXQanalyze, DXQplant.analytics detects irregularities in the painting quality and meticulously identifies possible causes. This allows to increase the first-run rate and thus the overall equipment effectiveness.
The software products of the Digital Factory, united under the name DXQ, are divided into four product families: DXQanalyze, DXQoperate, DXQcontrol and DXQsupport. In this article we would like to introduce you to the two analysis tools DXQequipment.analytics and DXQplant.analytics of the DXQanalyze product family.
DXQequipment.analytics
You may already know the analysis tool → DXQequipment.analytics from our article "Smart paint shop with DXQequipment.analytics" published in March 2019.
This tool helps to collect, evaluate and visualize robot and process data. The software seamlessly records all data from the painting process and analyzes it. Based on this, a ‘digital fingerprint’ is created for each painted car body. Learn more about the use of DXQequipment.analytics in the painting process in our article "Smart Paint Shops with DXQequipment.analytics".
DXQequipment.analytics for oven
The analysis software DXQequipment.analytics shown above has been extended by a new module. It can now be used for the first time in the drying process.
The software DXQequipment.analytics for oven is an Industrial Internet of Things (IIoT) solution, which simulates body heating curves in the oven in real time. This means that paint shop operators can see the current temperature profiles directly on the workpiece from the current drying process and additionally get various body-related characteristic values. This enables the conditions in the oven, which are influenced by a variety of parameters, to be traced back precisely for each individual body. Deviations from the ideal process can then be identified at an early stage, and appropriate countermeasures put in place.
How does DXQequipment.analytics for oven work?
The software’s algorithm calculates the heating curves at different measuring points on the body based on the current system parameters. An optical measurement inside the oven, which serves as a reference point, ensures the quality of the calculated profiles in the long term. Each body gets its own electronic workpiece data record where all the data is stored. In addition, all physically measured data from test runs is stored centrally and used to train the algorithm.
How does DXQequipment.analytics for oven contribute to quality improvement?
In order to guarantee a consistently high quality of the painting result, the specific heating behavior for each body type must be scrupulously observed. Up to now, the processes were adjusted based on data from the initial calibration runs during commissioning and from test runs conducted at intervals of several weeks during production. In the interim, the operator had no information about the actual heating behavior on the body. With DXQequipment.analytics we can now close this information gap and contribute to quality improvement.
With DXQequipment.analytics for oven, we place the focus on the quality of plant operation. The real-time simulation of heating curves, a clear visualization, and the central, continuous data storage greatly increase transparency for the operator. At the same time, they serve as an individual quality certificate for the entire drying process for each individual body. The tool uses the body-specific data and relates them to quality data generated during the inspection of the end product. This allows to determine which causes in the process influence the quality of the end product. DXQequipment.analytics for ovens also provides an important component for the Dürr tool DXQplant.analytics by storing body-specific data.
DXQplant.analytics
Our third software of the DXQanalyze family is DXQplant.analytics. On the way through a paint shop, each body undergoes different complex process steps. The basic concept of DXQplant.analytics is to save information from different sources via the industrial Internet of Things (IIoT) specifically for each individual body in a whole-of-life record, compare this information with quality data, and statistically evaluate the data of all the vehicles produced. Its goal is to determine the normal behaviour of the plant with its process steps from the collected data sets. Artificial intelligence is used to identify patterns, compare them with quality data, and derive consequences for a targeted optimization of the first-run rate.
How does DXQplant.analytics work?
DXQplant.analytics uses big data models to identify previously unknown interrelationships in the paint shop. The infrastructure for the digital data flows is the IIoT platform ADAMOS, which is used to collect all production, process, and quality data. The software DXQplant.analytics determines subsequently the quality condition from this information and visualizes it on a dashboard for the paint shop operator. Our customers can find a quick overview of quality-related performance indicators, such as the production rate including post-processing and the second-run rate. The performance indicators are automatically associated with the recorded quality defects and evaluated on the basis of the defect cause. The paint shop operator is shown a visualization of where quality defects occur more frequently on the bodies, and in this way is helped to find production vulnerabilities.
Our DXQplant.analytics software enables our customers to continuously optimize their plant and thus increase the first-run rate in the long term.
How does DXQplant.analytics contribute to quality improvement?
DXQplant.analytics uses artificial intelligence to identify systematically occurring quality deviations and uncover their causes in the process. With the help of self-learning data models, recurring patterns in recorded quality data are determined and linked to anomalies in the production process. Up to now, defects were often only found during the visual inspection, meaning that bodies had to be reworked. In contrast, DXQplant.analytics uses the historical data to determine that the defect is not sporadic but rather a systematic defect pattern and provides advance information about impending quality problems. With this tool we can considerably improve the quality management in paint shops and production plants.
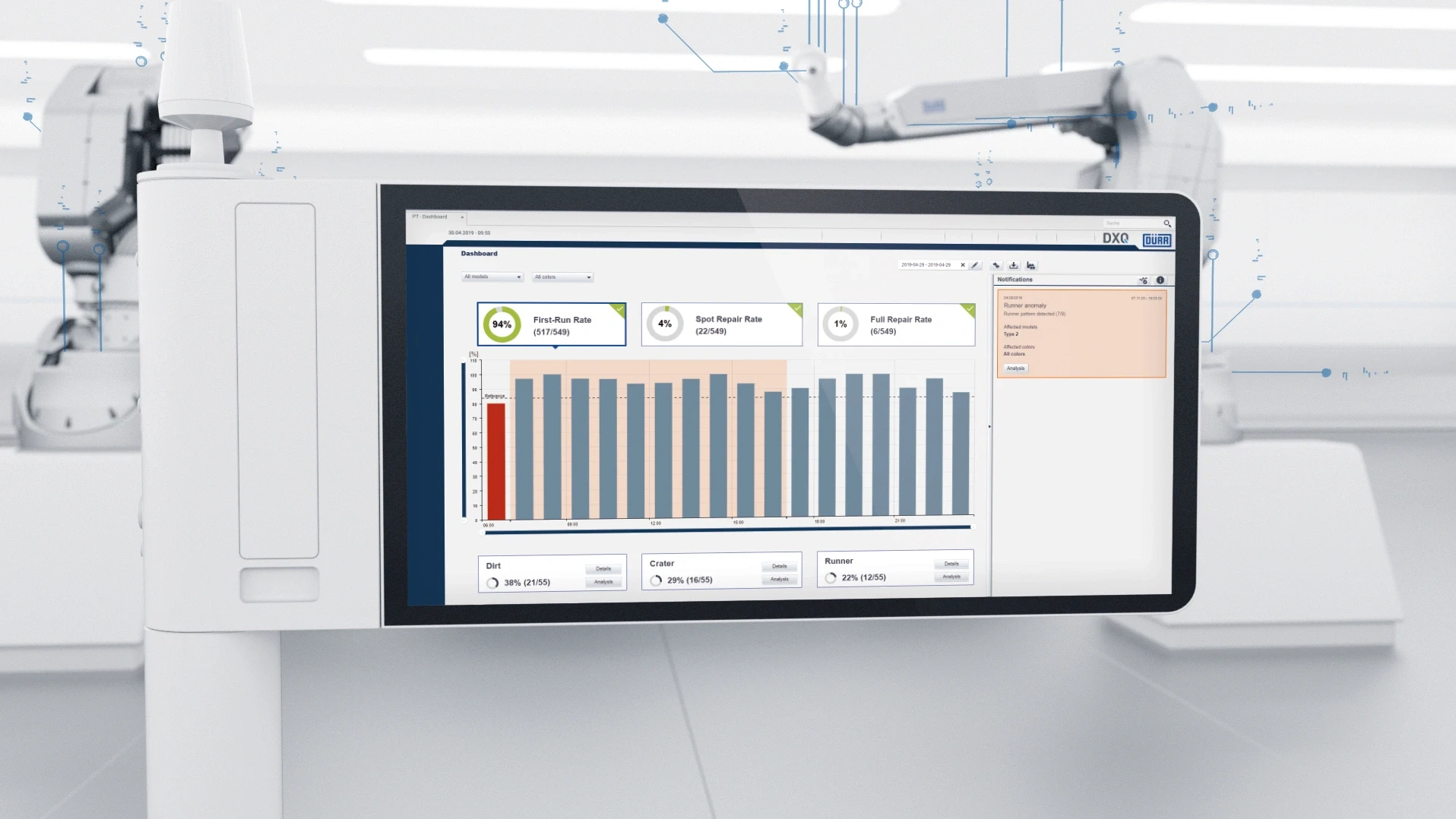
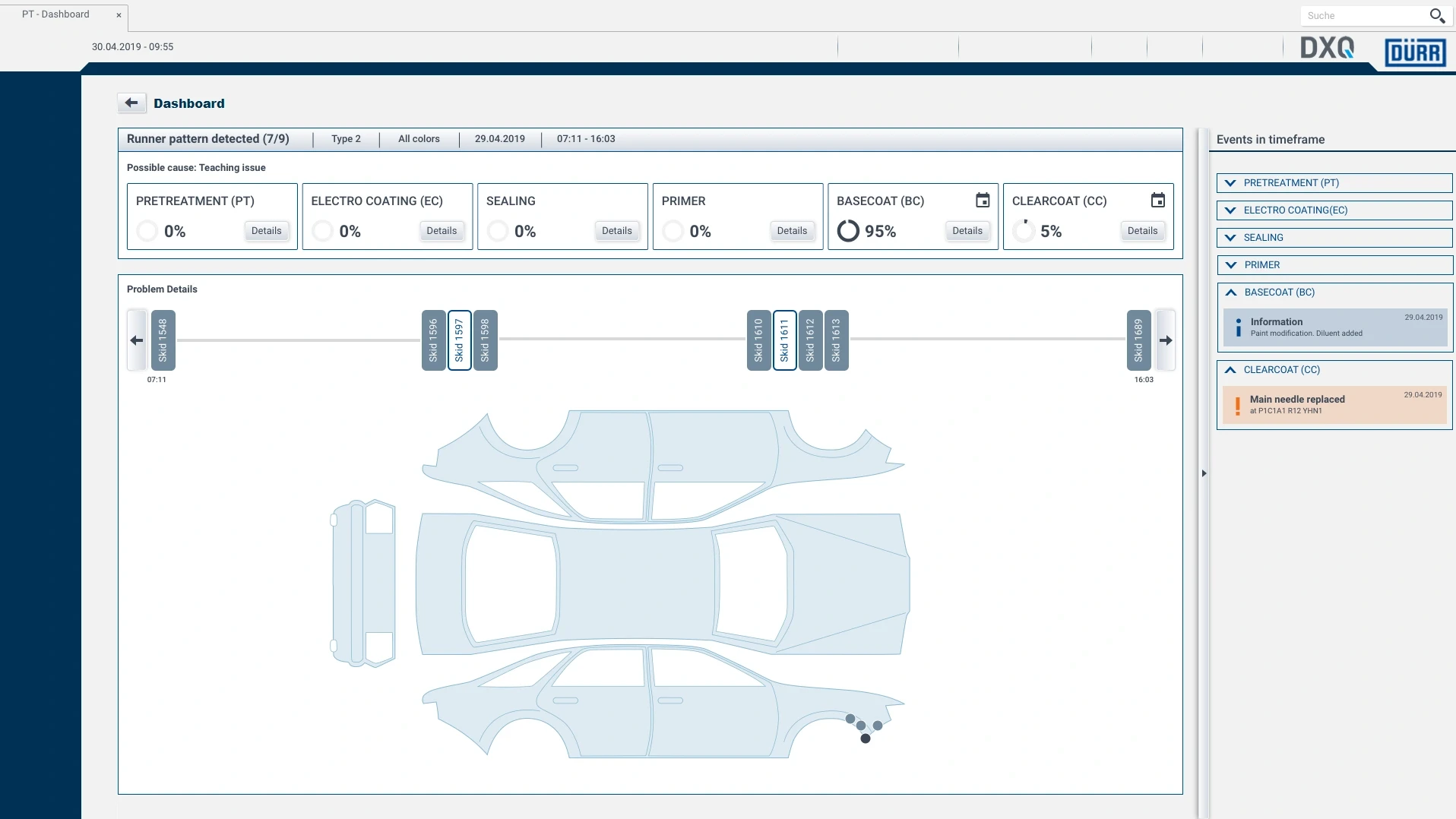